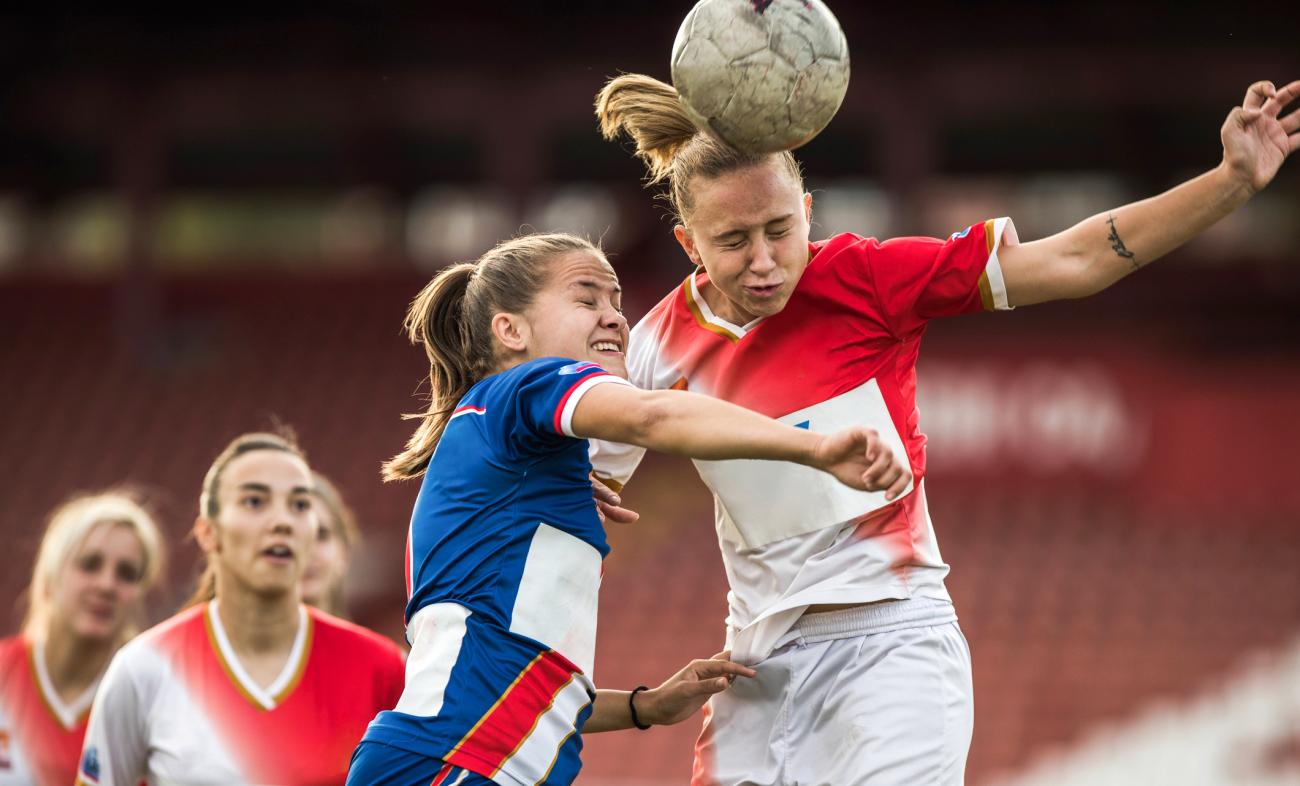
Using computer software to track headers in soccer game video footage could lead to the widespread collection of head impact data.
Sport-related head injury is a significant health concern that can lead to harmful effects down the line, such as permanent brain injury. Research led by Vancouver Coastal Health Research Institute researcher Dr. Lyndia Wu and Ahmad Rezaei used a novel computer vision algorithm to create a head injury baseline that could help protect sports participants from short- and long-term negative outcomes from head impacts.
Published in the journal Nature’s Scientific Reports, their video analysis software was able to identify soccer headers in pre-recorded soccer game footage.
“To our knowledge, this is the first paper to use this video-based learning approach to detect heading exposure in soccer,” notes Wu. “It is a relatively novel contribution that is shifting away from conventional thinking about how to track possible head injuries, such as concussion.”
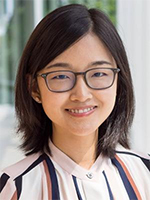
Heading is a common technique that soccer players use to redirect the ball during game play. Headers represent approximately 90 per cent of all head impacts in soccer, with the remainder being mostly unintentional player-to-player or head-to-ground impacts, says Rezaei.
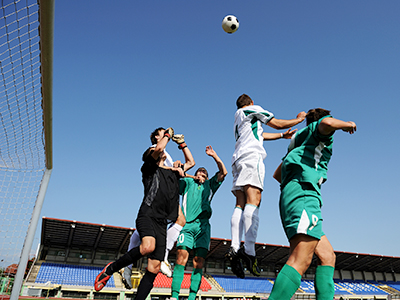
Head injuries are any type of trauma to the brain, skull or scalp. One type of acute head injury is concussion, which occurs when the soft tissues of the brain that are normally protected by the skull and cerebrospinal fluid undergo substantial tissue stretch due to excessive head accelerations. Concussion can occur as a result of a fall or any injury that rapidly shakes the brain inside the skull.
Successive or highly severe head injuries have been linked with an increased risk of long-term brain effects, such as headaches and difficulties balancing, concentrating and remembering. However, there is currently a gap in scientific knowledge about what types of head injuries can lead to long-term brain health consequences.
Computer vision algorithm outperformed wearable technology in training sessions
Wu and Rezaei’s computer vision algorithm used deep learning convolutional neural networks (CNNs) to track when a soccer ball made contact with a soccer player’s head.
Deep learning is a computer algorithm that tries to mimic the human brain’s ability to analyze data and make deductions or predictions. CNNs are a type of deep learning often used to identify and classify types of images, in this case, soccer headers.
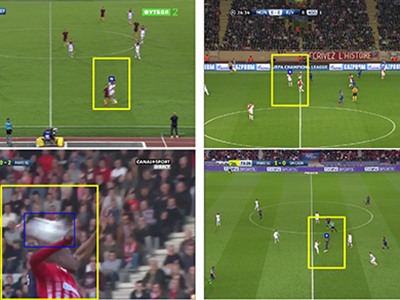
Wu and Rezaei’s video analysis CNN was trained to estimate the position of the ball in matches, and then crop and zoom into the frame to improve ball detection accuracy.
Their algorithm detected over 95 per cent of headers in training sessions and 92.9 per cent in five independent full soccer games — with an average of eight missed headers per game. The software performed less well in an independent, full soccer game test, with precision dropping to 21.1 per cent and an average of 379 false-positive headers per game.
“This difference likely had to do with the training session having too few non-headers versus headers,” notes Rezaei. “While the training videos averaged seven non-headers per each header, the full-game test averaged 110 non-headers for every header.”
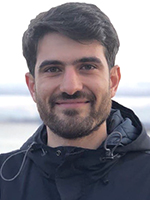
Previous research into headers has often used wearable technology, such as a mouthguard, to detect head impacts. In comparison, Wu and Rezaei’s video analysis CNN had similar or higher accuracy, states Rezaei.
Header analysis using Wu and Rezaei’s CNN technology also took a fraction of the time that it would take for humans to perform a similar analysis, explains Wu. “In a previous study, 14 trained reviewers analyzed around 160 hours of video to capture around 200 headers,” she says. “Our software could complete a similar analysis in a matter of minutes.”
As an added advantage, because the platform is built on open-source software, it would come at a fraction of the cost.
Data could support health care providers in preventing harm from head injuries
Wu and Rezaei’s study paves the way for the collection of both quantitative and qualitative data that could help analyze nuances of head injury in sport. For example, linking header frequency with medical data could determine whether a certain number of headers experienced by any individual can predispose them to negative brain changes in the future.
Coupled with wearable technology, Wu and Rezaei’s software could also provide more robust data-sets related to head impacts.
“This is the first step towards building large, video-based head impact exposure data-sets from sports footage so that we can better understand how past heading exposure relates to long-term cognitive outcomes,” says Wu.
“In the future, such algorithms can be developed into a potentially real-time exposure tracking algorithm that would help us with individualized exposure monitoring and tracking.”
“This has the potential to become a sideline tool that health care providers, as well as coaches and sports medicine staff, use to help limit and prevent potentially damaging head injury.”