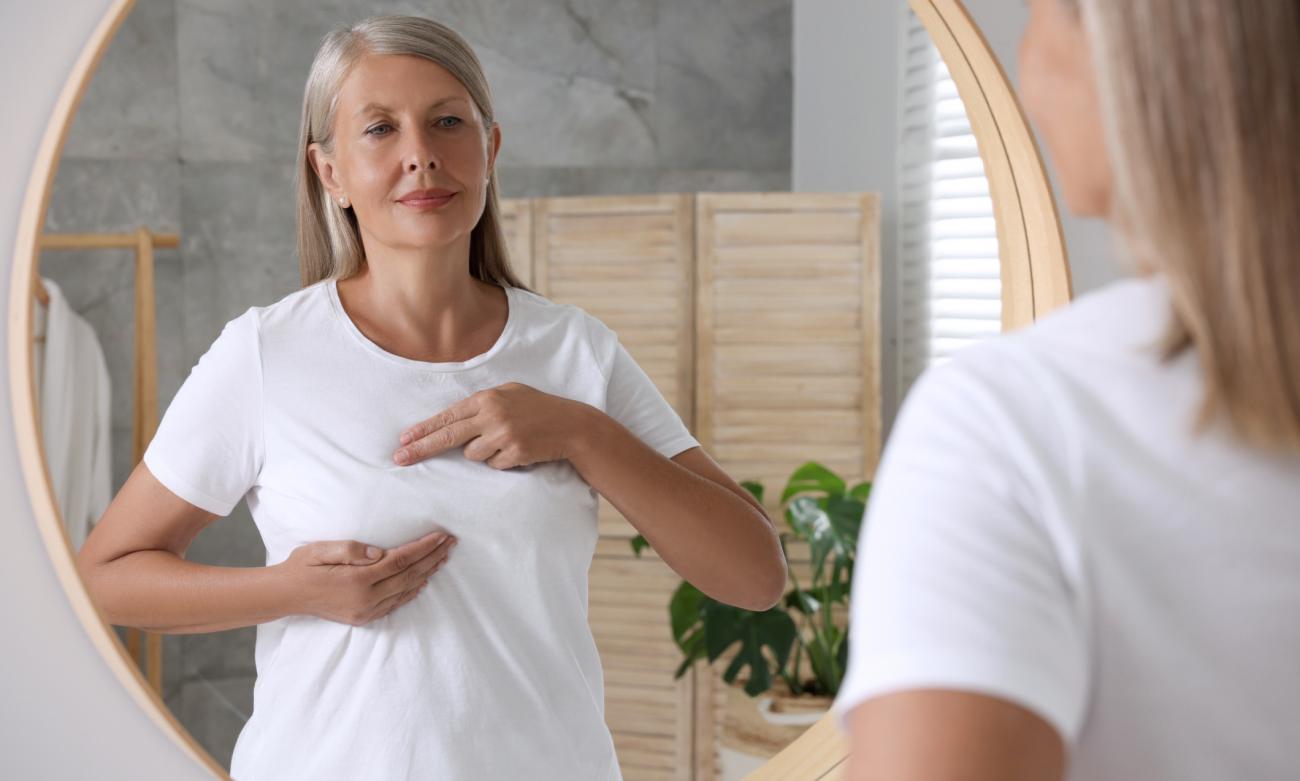
The powerful machine learning algorithm can predict the need for post-mastectomy radiotherapy in 12 questions.
Researchers are leveraging the power of machine learning to support breast reconstruction decision-making among breast cancer patients. A breakthrough study, published in the journal Plastic and Reconstructive Surgery: Global Open, converts data on breast reconstruction outcomes following a mastectomy, which involves the removal of all or part of the breast, into a simple-to-use online tool to guide patient care discussions.
Led by Dr. Kathryn Isaac, a Vancouver Coastal Health Research Institute researcher, the study outlines a predictive modelling approach that calculates the odds of a patient requiring radiotherapy after receiving breast reconstructive surgery immediately following a mastectomy. An important decision for a patient, the timing and type of breast reconstruction surgery can have varying health and aesthetic outcomes.
“The tool that we developed can be tailored to meet the individual needs of breast cancer patients.”
Many breast cancer patients who undergo a mastectomy will require post-surgical radiation therapy, also called radiotherapy: the administration of high doses of radiation to kill cancer cells or shrink tumours. Many patients will also request immediate or delayed breast reconstruction surgery.
Autologous breast reconstruction involves transferring skin from the patient’s body to recreate the breast silhouette. Alloplastic breast reconstruction uses synthetic materials, such as silicone.
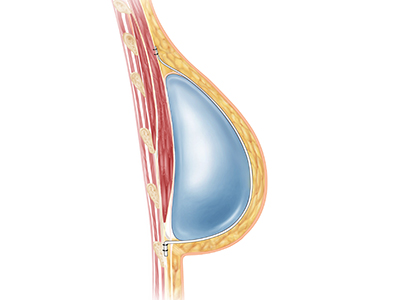
There is reduced scarring and fibrosis of the breast skin following radiation therapy in patients who receive autologous breast reconstruction, notes Isaac. In alloplastic procedures, the body creates a fibrous scar tissue capsule around the synthetic implant, which has an approximate 30 to 40 per cent likelihood of tightening following radiation therapy. Called capsular contracture, the condition can lead to breast distortion, discomfort or pain that can become chronic.
“For some patients, capsular contracture can feel like having a tight band around their chest,” Isaac shares. “It can be very debilitating, and can lead to an implant that is contracted and abnormally high on the body.”
A dozen questions to deepen patient understanding of care options
Isaac’s study involved a chart review of 800 breast cancer patients who underwent both alloplastic and autologous breast reconstruction surgery at the same time as receiving a mastectomy. Of these, 325 patients were later recommended to undergo radiotherapy to prevent the return of their cancer.
Using a machine learning computer algorithm, Isaac and her team analyzed patient information to triangulate patient disease and demographic characteristics with a clinical recommendation of radiation therapy post-mastectomy. Following several years of data analysis, the team identified a single computer algorithm, called elastic net, that was able to narrow down the predictive preoperative factors of post-mastectomy radiotherapy to 12, such as age at diagnosis and tumour size.
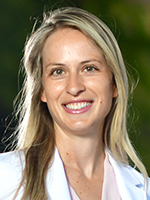
Isaac and her team then transformed these 12 predictive factors to assess a patient’s odds of needing post-mastectomy radiotherapy into an online calculator. The calculator can be used by clinicians together with breast cancer patients when discussing care options and patient preferences. For example, a clinician would enter patient data into the tool, which would then provide a prediction of the likelihood of the patient requiring radiotherapy following a mastectomy to treat their breast cancer.
“Each patient will have varying levels of risk and potential complications based on their breast cancer, body, age, desires, circumstances and various other factors,” notes Isaac.
“What is most important is that patients have access to as much clinically relevant information as possible to make the best decision for them.”
Isaac is pursuing additional research into the application of the tool in clinical settings at UBC, the University of Calgary and the University of Western Ontario, with hopes to make the tool more widely accessible.