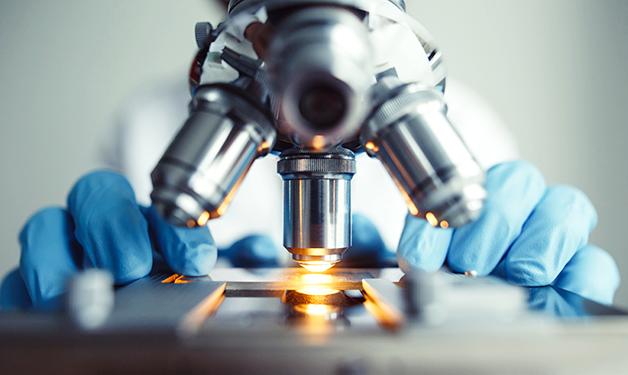
Computers enhance traditional diagnosis, but more research is needed.
Inside the Department of Pathology and Laboratory Medicine at Vancouver General Hospital (VGH), a clinician peers through a microscope. She is looking at a thin slice from a block of paraffin wax embedded with tissue samples, searching for clues to cure disease. VGH neuropathologist and VCHRI researcher Dr. Stephen Yip calls this the analogue version of histology—the study of the structure of tissues at the microscopic level. Research he and colleagues are conducting is paving the way for histology 2.0 in the age of digital technology.
Yip and Adrian Levine, a resident of pathology and laboratory medicine at VGH, are researching how software programs that use deep learning can add a layer of quality control to diagnosing diseases from tissue samples. Deep learning here refers to computer software that uses algorithms to recognize what data represents, such as curves and lines in an image. This is similar to technology found in self-driving cars, which uses machine learning to detect features in the landscape.
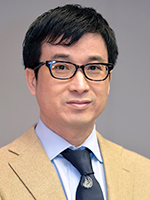
The deep learning tools Yip and Levine are using have been around for a decade, but only in the past few years have they become popular for diagnosing diseases, such as cancer, from tissue samples. Advances in an area of deep learning called convolutional neural networks (CNNs) have been pivotal to this newfound application.
CNNs are computer algorithms that identify specific characteristics in images using a layering technique. This makes CNNs ideal for medical specialties that rely heavily on image-based data—such as radiology and pathology—for interpreting information and making diagnoses. They enable computers to accurately differentiate between, for example, images of cancerous and non-cancerous cells.
Research is fueling the new frontier of disease identification
To make it possible for computers to identify disease, millions of images of tissue samples need to be uploaded as digital copies. These images train the deep learning software to identify different medical conditions based on previous diagnoses.
“The gold standard will be to make sure the software works on new real-world tissue samples.”
While all the images in the database provide essential baseline information, the long-term hope is for this technology to be able to identify diseases from images it has never seen before.
At some centres, radiologists already apply machine learning as part of their day-to-day work, including for examining X-rays and CT scans. By comparison, histologists have been peering through microscopes at tissue samples sandwiched between panes of glass since the mid-1800s, which Yip calls the “analog” way of doing things in the new digital frontier.
“We still have to digitize a huge number of slides to provide the training data our software needs to reach the gold standard of disease identification.”
Research, including a paper submitted by Levine, Yip and colleagues called “Machine learning in pathology: a primer on techniques and applications,” discusses examples where combining CNNs with a pathologist’s diagnosis improved the accuracy of the diagnosis by several percentage points. In one example, the CNN was able to more accurately distinguish between different types of lung cancer than its human pathologist counterpart.
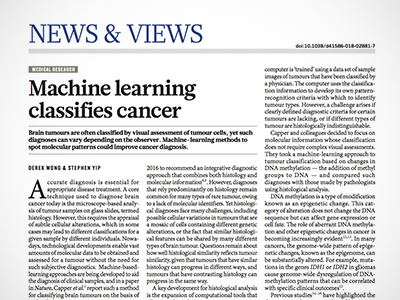
CNN technology is still in the early development stages as a clinical diagnostic tool. Scientists do not yet know with 100 per cent certainty whether there may be inherent biases in its programming that could interfere with a proper diagnosis. In other words, at least for the time being, diagnoses will still need to be signed off by a qualified human.
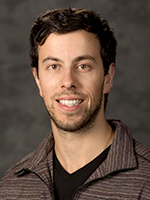