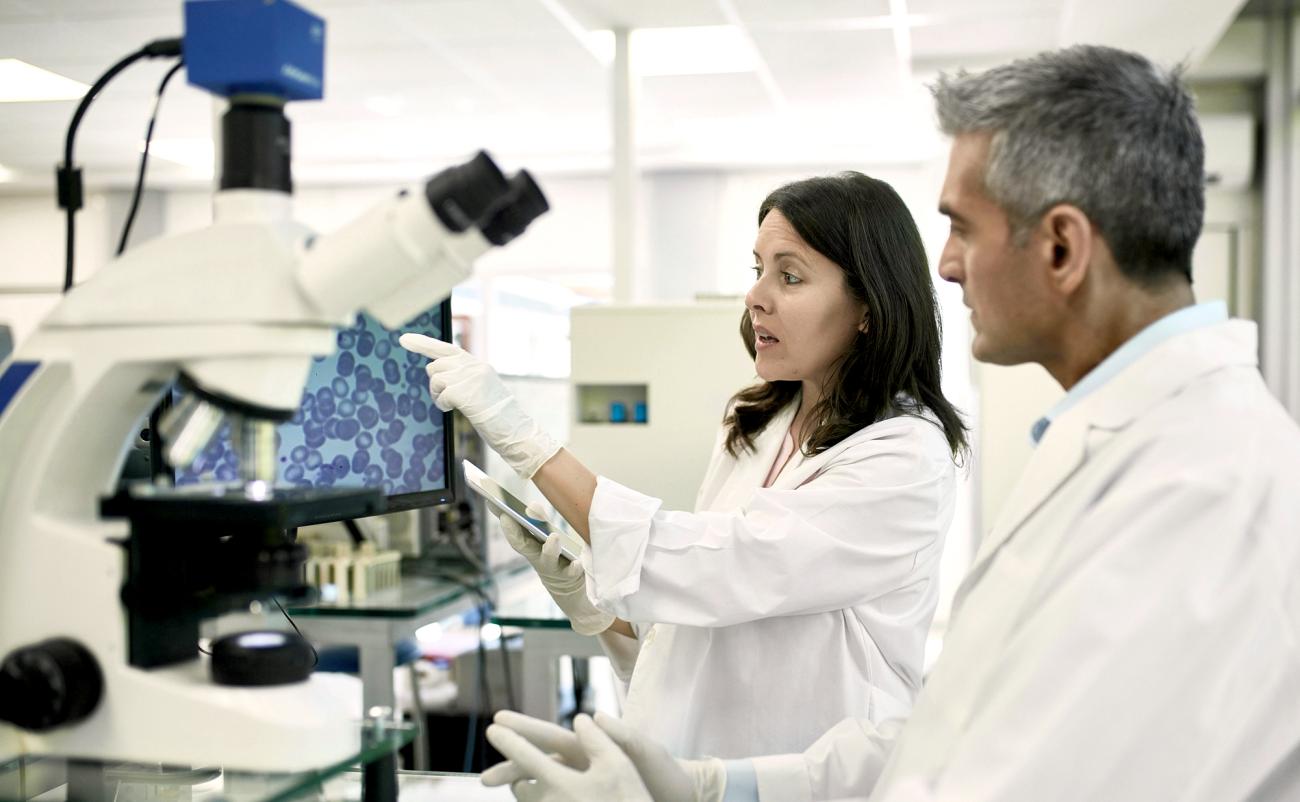
Artificial intelligence algorithms could provide added support to remote communities and other areas that lack available specialists.
Ovarian cancer impacts over 3,100 Canadian women each year, making it the most lethal of all female reproductive cancers. A new study led by Vancouver Coastal Health Research Institute researcher Dr. Ali Bashashati is using artificial intelligence (AI) to aid pathologists in the diagnosis of ovarian cancer to improve patient outcomes.
Published in Modern Pathology in September 2022, Bashashati’s research builds on the understanding that ovarian cancer is not a single disease, but several distinct subtypes, called histotypes.
Bashashati and his team compared ovarian cancer disease classifications made by an AI machine learning-based model against those of a team of expert gynecologic pathologists who specialize in the diagnosis of female reproductive cancers.
Using a cohort of 948 ovarian cancer tissue specimens from Vancouver General Hospital, Bashashati’s team developed a series of AI computer algorithms that can identify four histotypes of ovarian cancer with a high degree of accuracy.
“To the best of our knowledge, our AI model is the first of its kind to achieve the highest performance for ovarian carcinoma histotype diagnosis, approaching the performance level of expert gynecologic pathologists,” says Bashashati.
Each ovarian cancer histotype has unique characteristics and telltale signs that can nonetheless be difficult to identify in some cases.
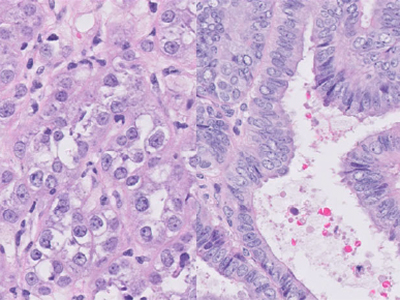
Prior research has shown that general pathologists have weak to moderate interobserver agreement — the extent to which two or more experts agree — when it comes to ovarian cancer histotype classification, says Bashashati. Gynecologic pathologists, on the other hand, have strong to very accurate interobserver agreement.
Providing general pathologists with additional tools to achieve a higher interobserver agreement would support optimal diagnostics and the timeliest and best possible treatment approach.
Advanced technology to extend the reach of specialist care
The AI algorithms Bashashati and his team developed were convolutional neural networks (CNNs) — highly advanced technology that attempts to mimic complex neurological connections in the human brain that support such thought processes as pattern recognition and deductive reasoning.
The research team’s CNNs overcame some of the challenges other similar CNNs previously faced, such as distinguishing between colours and other characteristics in tissue sample slides.
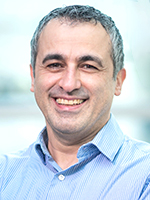
“When we tested our best-performing AI model using another cohort of 60 patients from a different Canadian hospital, the algorithm correctly diagnosed 52 of the cases,” says Bashashati.
Expert gynecologic pathologists re-reviewed eight cases that were misclassified by the AI algorithm. In four of the eight cases, the experts ended up agreeing with the AI classification.
“This means that the accuracy of our best-performing AI model approached 93 per cent, which is incredibly exciting.”
Bashashati’s CNNs were designed to be transferable AI classification aids that could be used at various hospitals.
“Many community hospitals do not have a gynecologic pathologist on staff to assist a general pathologist with the classification of an ovarian cancer subtype,” says Bashashati. “As such, there is a great need to provide general pathologists with additional tools to aid them in ovarian cancer diagnosis, particularly given increased demand for specialists in remote communities and a limited number of available gynecologic pathologists across British Columbia.”
"Our AI models may provide a separate set of artificial eyes during clinical assessments to improve diagnostic performance when used alongside the assessment of a pathologist.”
Bashashati is now working to further validate these findings using an international cohort of 8,000 ovarian cancer tissue sample slides.
This research was supported by philanthropic funding from the Carraresi Foundation and Toigo Family through the VGH & UBC Hospital Foundation, along with a Canadian Institutes of Health Research grant.