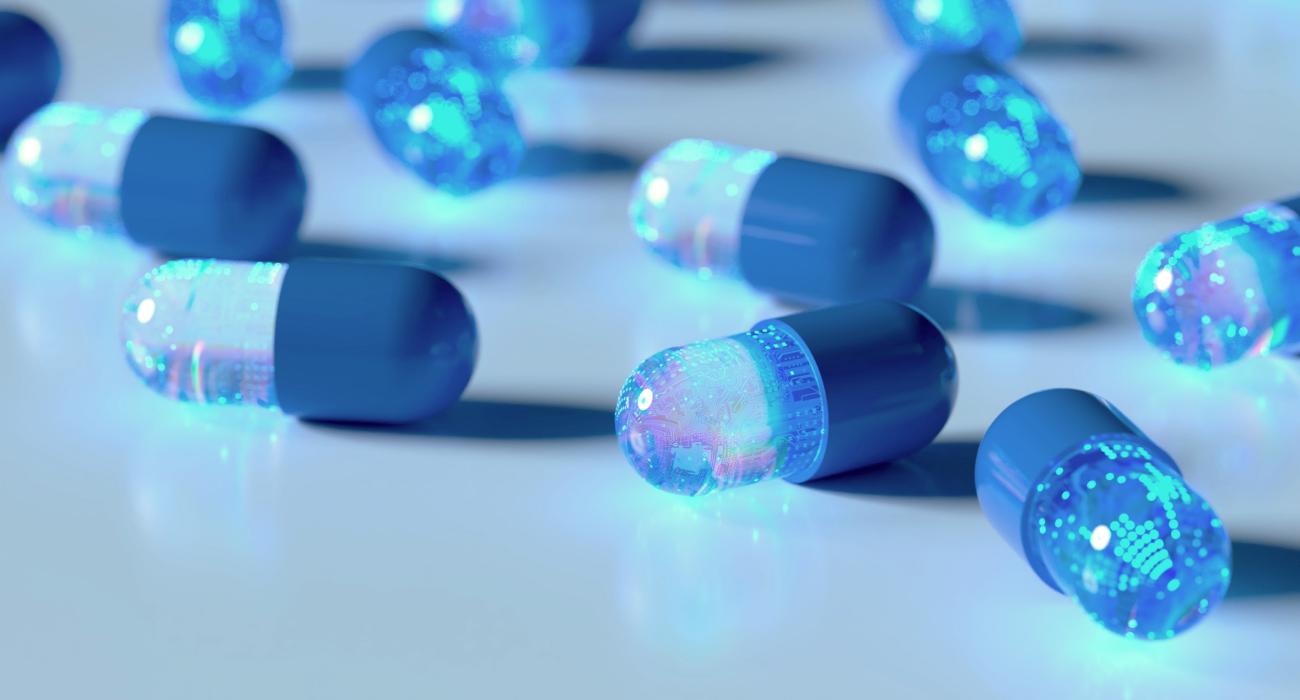
A world leader in AI and other computational methods in health research, Dr. Artem Cherkasov placed first among competitors in two recent CACHE Challenges.
Vancouver Prostate Centre researcher Dr. Artem Cherkasov recently won two competitions in the international CACHE Challenges, leveraging advanced computational methods and artificial intelligence (AI) to find new potential treatment avenues for Parkinson’s disease and COVID-19. Cherkasov’s submissions topped results from dozens of other researchers from leading academic, industry and non-profit sector institutions around the world.
"The Critical Assessment of Computation Hit-finding Experiments (CACHE) Challenges are a unique opportunity to make breakthroughs in early drug discovery.”
CACHE Challenges are stewarded by the Canadian-based non-profit, Conscience, formed in partnership with the Structural Genomics Consortium. Conscience is headed by leading academics, researchers, industry representatives and community members.
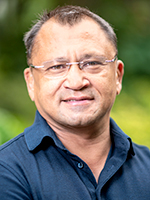
A select number of entrants to the CACHE Challenges are chosen for each competition. Entrants engage in hit-finding, a process critical to the drug discovery pipeline in which researchers predict which molecules will bind to a predefined target on a disease to potentially disable it. Predictions are evaluated experimentally in a state-of-the-art, Structural Genomics Consortium laboratory, with results and all chemical structures identified by entrants released to the public for free.
“To participate in two CACHE challenges and to win both was a validation of our research approaches and methods in the growing field of computational and AI-powered drug discovery,” says Cherkasov. “There are still countless diseases in need of more or any effective treatments that could benefit from similar approaches.”
The potential of AI to accelerate novel treatments for diseases
The first CACHE competition that Cherkasov participated in, Challenge #1, invited researchers to identify molecular ‘hits’ to target the WD40 repeat (WDR) domain of the leucine-rich repeat kinase 2 (LRRK2) protein — a therapeutic target associated with Parkinson’s disease.
“There is presently no cure for Parkinson’s disease, making drug discovery in this area of extreme importance to the care of patients and to their families.”
In collaboration with researchers from Carnegie Mellon University (CMU), Cherkasov and other members of the UBC team used the Deep Docking platform to identify two series of candidate drugs for Parkinson’s. Deep Docking is an AI-powered approach developed by Cherkasov and his research team that enables scientists to search through billions of molecules for candidate drugs — a task not amenable to traditional methods.
For the CACHE Challenge #1, the UBC/CMU team leveraged Deep Docking to screen 4.5 billion small molecules, narrowing them down to a handful of candidates. Fourteen of these were experimentally validated, contributing to the team’s top score among all participants.
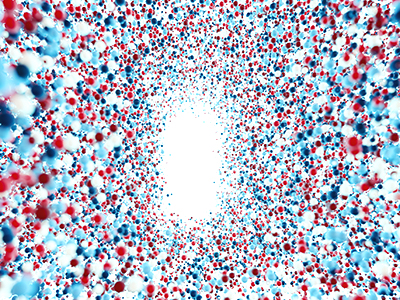
Cherkasov’s group also participated in and won the third CACHE competition, Challenge #3, against 23 teams from 11 countries. Teams were tasked with identifying inhibitors for the adenosine diphosphate ribose binding site of the macrodomain 1 (mac1) of SARS-CoV-2 non-structural protein 3. The COVID-19 virus uses mac1 to evade the immune system of its host, and no effective treatment has been discovered to impede that activity.
“The democratization of drug discovery requires the establishment of AI-based pipelines that are orders of magnitudes faster and cheaper. The CACHE Challenges are contributing to this goal.”
Together with Dr. Francesco Gentile — an assistant professor in the Faculty of Science at the University of Ottawa and a former Cherkasov’s postdoc — Cherkasov employed an array of AI tools, including Deep Docking, to screen an ultra-large chemical library. The team identified 83 candidate compounds for their submission, and a number of them demonstrated significant efficacy against the SARS-2-CoV virus.
“At some point or other during our lifetimes, many of us may need drugs currently unavailable,” Cherkasov states. “We need to accelerate drug design using the processing power of AI to get these medications to market sooner rather than later.”